成長/個性化第一天/結構化任務/添加鏈接
Add a link
![]() Suggest potential wikilinks as easy edits for newcomers
|
本頁描述了Growth team在“添加鏈接”結構化任務上的工作,這是Growth團隊通過newcomer提供的一種結構化任務主頁。 此頁麵包含主要資產、設計、開放性問題和決策。 大多数渐增式的进展更新會发布在一般的增长团队最新進展页面上,而一些大型或详细的最新進展会发布在这里。
截至2021年8月,該任務的第一次迭代已部署到以阿拉伯語、捷克語、越南語、孟加拉語、波蘭語、法語、俄語、羅馬尼亞語、匈牙利語和波斯語維基百科創建的所有新帳戶的一半。 我們已經分析了前兩週的數據功能的部署,我們發現新人進行了很多這樣的編輯,而且他們的回复率很低。 從這一分析中吸取的教訓促使我們對該功能進行了改進,結果鼓勵我們將該功能的部署範圍擴大到更多wiki。
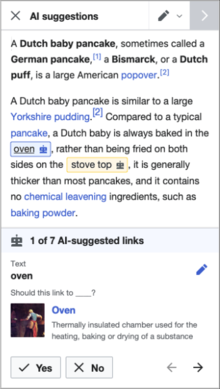
您可以在這些交互式原型中看到我們正在構建的內容。 請注意,因為它們是原型,並非所有按鈕都有效:
團隊成員在Wikimania 2021上介紹了這項工作的背景、算法、實現和結果。 在這裡觀看視頻。 and the 幻燈片在這裡。
当前状态
- 2020-01-07: 鏈接推薦算法可行性初評
- 2020-02-24: 改進鏈接推薦算法的評價
- 2020-05-11: 關於結構化任務和鏈接建議的社區討論
- 2020-05-29: 初始線框
- 2020-08-27: 後端工程開始
- 2020-09-07: 移動設計的第一輪用戶測試
- 2020-09-08: 呼籲社區討論最新設計
- 2020-10-19: 移動設計的第二輪用戶測試
- 2020-10-21: 桌面設計的第一輪用戶測試
- 2020-10-29: 前端工程開始
- 2020-11-02: 第二輪用戶測試桌面設計
- 2020-11-10: 徵集阿拉伯、越南和捷克社區對設計的反饋
- 2021-04-19: 添加了術語和測量部分
- 2021-05-10: 該功能正在我們的四個試點wiki上進行生產測試
- 2021-05-27: 部署到阿拉伯語、越南語、捷克語和孟加拉語維基百科的一半新人
- 2021-07-21: 中文
- 2021-07-23: 發布了功能部署前兩週的分析。
- 2021-08-15: Wikimania上的演示關於背景、實現、算法和結果。
- 2022-05-20: Completion of Iteration 2 of the feature, which includes improvements based on community feedback and data analysis (see list of improvements on the Add a link "Iteration 2" page.
- 2022-06-19: Publish "add a link" Experiment Analysis
- 2022-08-20: start work to address patroller feedback about structured tasks (T315732)
- 2022-09-02: publish Newcomer task edit type analysis
- 2023-12-31: Almost all Wikipedias now have this task available for newcomers. The exception are a dozen of small wikis where there are not enough articles to activate the algorithm, and English and German Wikipedias.
- 下一步: deployments to all wikis and work with patrollers to improve their experience dealing with link edits.
概述
結構化任務旨在將編輯任務分解為對新人有意義且在移動設備上有意義的分步工作流程。 Growth團隊相信,引入這些新的編輯工作流程將使更多的新人開始參與維基百科,其中一些人將學習進行更實質性的編輯並參與到他們的社區中。 在與社區討論結構化任務的想法之後,我們決定構建第一個結構化任務:“添加鏈接”。 此任務將使用算法指出可能是好的wikilink的單詞或短語,新人可以接受或拒絕這些建議。 通過這個項目,我們希望在這些問題上有所收穫:
- 結構化任務對新人有吸引力嗎?
- 新人在移動設備上完成結構化任務是否成功?
- 他們會產生有價值的編輯嗎?
- 他們是否會帶領一些新人增加他們的參與度?
After completion of "Add a link" Experiment Analysis, we can conclude that the "add a link" structured task improves outcomes for newcomers over both a control group that did not have access to the Growth features as well as the group that had the unstructured "add links" tasks, particularly when it comes to constructive (non-reverted) edits. The most important points are:
- Newcomers who get the Add a Link structured task are more likely to be activated (i.e. make a constructive first article edit).
- They are also more likely to be retained (i.e. come back and make another constructive article edit on a different day).
- The feature also increases edit volume (i.e. the number of constructive edits made across the first couple weeks), while at the same time improving edit quality (i.e. the likelihood that the newcomer's edits are reverted).
為什麼是維基鏈接?
下面摘自結構化任務頁面,解釋了為什麼我們選擇構建“添加鏈接”作為第一個結構化任務。
增長團隊目前(2020年5月)希望將“添加鏈接”工作流優先於上表中列出的其他工作流。 儘管其他工作流程,例如“copyedit”,似乎更有價值,但有一系列原因我們希望首先從“添加鏈接”開始:
- 在短期內,我們首先要做的最重要的事情是證明“結構化任務”可以工作的概念。 因此,我們希望構建最簡單的一個,以便我們可以部署到用戶並從中學習,而不必在第一個版本上投入太多。 如果第一個版本進展順利,那麼我們將有信心投資於更難構建的任務類型。
- “添加鏈接”似乎是我們構建的最簡單的方法,因為已經存在由WMF研究團隊構建的算法,該算法似乎在建議wikilinks方面做得很好(請參閱算法部分)。
- 添加維基鏈接通常不需要新人輸入他們自己的任何內容,我們認為這將使我們的設計和構建變得特別簡單 - 以及讓新人完成。
- 添加維基鏈接似乎是一個低風險的編輯。 換句話說,一篇文章的內容不會因為錯誤地添加鏈接而受到損害,就像通過錯誤地添加引用或圖像一樣。
设计
本節包含我們當前的設計思路。 要查看“添加鏈接”結構化任務的完整設計思路,請參閱 幻燈片,其中包含背景、用戶故事和初始設計概念。
我們的設計經過幾輪用戶測試和迭代演變而來。 截至2020年12月,我們已經確定了我們將為該功能的第一個版本設計的設計。 您可以在這些交互式原型中看到它們。 請注意,因為它們是原型,並非所有按鈕都有效:
對比評測
當我們設計一個功能時,我們會研究維基媒體世界之外的其他軟件平台中的類似功能。 這些是為準備Android的建議編輯功能而進行的比較評論中的一些亮點,它們仍然與我們的項目相關。
- Task types – are divided into five main types: Creating, Rating, Translating, Verifying content created by others (human or machine), and Fixing content created by others.
- Visual design & layout – incentivizing features (stats, leaderboards, etc) and onboarding is often very visually rich, compared to pared back, simple forms to complete short edits. Gratifying animations often compensate for lack of actual reward.
- Incentives – Most products offered intangible incentives grouped into: Awards and ranking (badges) for achieving set milestones, Personal pride and gratification (stats), or Unlocking features (access rights)
- Users motivations – those with more altruistic motivations (e.g., help others learn) are more likely to be incentivized by intangible incentives than those with self-interested motivations (e.g., career/financial benefits)
- Personalization/Customization – was used in some way on most apps reviewed. The most common customization was via surveys during account creation or before a task; and geolocalization used for system-based personalization.
- Guidance – Almost all products reviewed had at least basic guidance prior to task completion, most commonly introductory ‘tours’. In-context help was also provided in the form of instructional copy, tooltips, step-by-step flows, as well as offering feedback mechanisms (ask questions, submit feedback)
Initial wireframes
After organizing our thoughts and doing background research, the first visuals in the design process are "wireframes". These are simply meant to experiment and display some of the ideas we think could work well in a structured task workflow. For full context around these wireframes, see the design brief slideshow.
-
Giving more explanation the first time the newcomer uses the workflow than on subsequent times
-
Explaining why the task is worth doing
-
Teaching concepts through checklists
-
Letting users disagree with algorithms and generate useful data in the process
-
Encouraging the newcomer to move on to more impactful tasks and learn more
-
Providing opportunities to learn the more powerful editing tools
-
Guardrails against low-quality contributions
-
Rewarding good work
移動模型:2020年8月
我們的團隊討論了上一節中的線框圖。 我們考慮了對新人來說什麼是最好的,考慮到社區成員表達的偏好,並考慮了工程限制。 2020年8月,我們採取了創建模型的下一步,旨在更詳細地展示該功能的外觀。 這些模型(或類似版本)將用於團隊討論、社區討論和用戶測試。 我們在使用這些模型時考慮的最重要的事情之一是我們在討論期間一直從社區成員那裡聽到的擔憂:結構化任務可能是向新人介紹編輯的好方法,但我們也希望如果他們感興趣,確保他們可以找到並使用傳統的編輯界面。
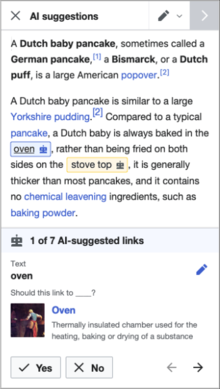
我們有兩種不同設計概念的模型。 我們不一定要選擇一種設計理念或另一種。 相反,這兩個概念旨在展示不同的方法。 我們的最終設計可能包含這兩個概念中的最佳元素:
- Concept A:結構化任務編輯發生在可視化編輯器中。 用戶可以看到整篇文章,並從“推薦模式”切換到源代碼或可視化編輯器模式。 不太關注添加鏈接,但更容易訪問可視化和源代碼編輯器。
- Concept B:結構化任務編輯發生在它自己的新區域。 用戶只會看到文章中需要他們注意的段落,如果他們願意,可以去編輯文章。 添加鏈接的干擾更少,但對可視化和源代碼編輯器的訪問更遠。
請注意,這組模型的重點是用戶流程和體驗,而不是文字和語言。 我們的團隊將通過一個過程來確定在功能中寫入文字的最佳方式,並向用戶解釋是否應該添加鏈接。

Static mockups
要查看這些設計概念,我們建議查看下面的全套幻燈片。

Interactive prototypes
You can also try out the "interactive prototypes" that we're using for live user tests. These prototypes, for Concept A and for Concept B, show what it might feel like to use "add a link" on mobile. They work on desktop browsers and Android devices, but not iPhones. Note that not everything is clickable -- only the parts of the design that are important for the workflow.
基本問題
在討論這些設計時,我們的團隊希望就一系列基本問題提供意見:
- 是否應該在文章中進行編輯(更多上下文)?或者在這種類型的編輯中獲得專門的體驗(更專注,但使用編輯器的跳轉更大)?
- 如果有人想編輯鏈接目標或文本怎麼辦?我們應該阻止它還是讓他們去標準編輯器?這是向他們介紹可視化編輯器的機會嗎?
- 我們知道支持新人發現傳統編輯工具對我們來說至關重要。 但是我們什麼時候這樣做呢?我們是否在結構化任務體驗期間提醒用戶可以轉到編輯器?或者定期在完成里程碑,比如在他們完成一定數量的結構化任務之後?
- “bot”在這裡是正確的術語嗎?還有哪些其他選擇? “算法”、“計算機”、“自動”、“機器”等?”有什麼可以更好地幫助傳達機器推薦是錯誤的以及人工輸入的重要性?
Mobile user testing: September 2020
Background
During the week of September 7, 2020, we used usertesting.com to conduct 10 tests of the mobile interactive prototypes, 5 tests each of Concepts A and B, all in English. By comparing how users interact with the two different approaches at this early stage, we wanted to better understand whether one or the other is better at providing users with good understanding and ability to successfully complete structured tasks, and to set them up for other kinds of editing afterward. Specific questions we wanted to answer were:
- Do users understand how they are improving an article by adding wikilinks?
- Do users seem like they will want to cruise through a feed of link edits?
- Do users understand that they're being given algorithmic suggestions?
- Do users make better considerations on machine-suggested links when they have the full context of the article (like in Concept A)?
- Do users complete tasks more confidently and quickly in a focused UI (like in Concept B)?
- Do users feel like they can progress to other, non-structured tasks?
Key findings
- The users generally were able to exhibit good judgment for adding links. They understood that AI is fallible and that they have to think critically about the suggestions.
- While general understanding of what the task would be ("adding links") was low at first, they understood it well once they actually started doing the task. Understanding in Concept B was marginally lower.
- Concept B was not better at providing focus. The isolation of excerpts in many cases was mistaken for the whole article. There were also many misunderstandings in Concept B about whether the user would be seeing more suggestions for the same term, for the same article, or for different articles.
- Concept A better conveyed expectations on task length than Concept B. But the additional context of a whole article did not appear to be the primary factor of why.
- As participants proceed through several tasks, they become more focused on the specific link text and destination, and less on the article context. This seemed like it could lead to users making weak decisions, and this is a design challenge. This was true for both Concepts A and B.
- Almost every user intuitively knew they could exit from the suggestions and edit the article themselves by tapping the edit pencil.
- All users liked the option to view their edits once they finished, either to verify or admire them.
- “AI” was well understood as a concept and term. People knew the link suggestions came from AI, and generally preferred that term over other suggestions. This does not mean that the term will translate well to other languages.
- Copy and onboarding needs to be succinct and accessible in multiple points. Reading our instructions is important, but users tended not to read closely. This is a design challenge.
Outcome
- We want to build Concept A for mobile, but absorbing some of the best parts of Concept B's design. These are the reasons why:
- User tests did not show advantages to Concept B.
- Concept A gives more exposure to rest of editing experience.
- Concept A will be more easily adapted to an “entry point in reading experience”: in addition to users being able to find tasks in a feed on their homepage, perhaps we could let them check to see if suggestions are available on articles as they read them.
- Concept A was generally preferred by community members who commented on the designs, with the reason being that it seemed like it would help users understand how editing works in a broader sense.
- We still need to design and test for desktop.
Ideas
The team had these ideas from watching the user tests:
- Should we consider a “sandbox” version of the feature that lets users do a dry run through an article for which we know the “right” and “wrong” answers, and can then teach them along the way?
- Where and when should we put the clear door toward other kinds of editing? Should we have an explicit moment at the end of the flow that actively invites them copyedit or do another level task?
- It’s hard to explain the rules of adding a link before they try the task, because they don't have context. How might we show them the task a little bit, before they read the rules?
- Perhaps we could onboard the users in stages? First they learn a few of the rules, then they do some links, then we teach them a few more pointers, then they do more links?
- Should users have a cooling-off period after doing lots of suggestions really fast, where we wait for patrollers to catch up, so we can see if the user has been reverted?
Desktop mockups: October 2020
After designing, testing, and deciding on Concept A for mobile users, we moved on to thinking about desktop users. We again have the same question around Concepts A and B. The links below open interactive prototypes of each, which we are using for user testing.
- Concept A: the structured task takes place at the article, in the editor, using some of the existing visual editor components. This gives users greater exposure to the editing context and may make it more likely that they explore other kinds of editing tasks.
- Concept B: the structured task takes place on the newcomer homepage, essentially embedding the compact mobile experience into the page. Because the user doesn't have to leave the page, this may encourage them to complete more edits. They could also see their impact statistics increase as they edit.
We are user testing these designs during the week of October 23. See below for mockups showing the main interaction in each concept.
-
Mockup of Concept A
-
Mockup of Concept B
Outcome
The results of the desktop user tests led us to decide on Concept A for desktop for many of the same reasons we chose Concept A for mobile. The convenience and speed of Concept B did not outweigh the opportunity for Concept A to expose newcomers to more of the editing experience.
Terminology
"Add a link" is a feature in which human users interact with an algorithm. As such, it is important that user have a strong understanding that suggestions come from an algorithm and that they should be regarded with skepticism. In other words, we want the users to understand that their role is to evaluate the algorithm's suggestion and not to trust it to much. Terminology (i.e. the words we use to describe the algorithm) play an important role in building that understanding.
At first, we planned to use the terms "artificial intelligence" and "AI" to refer to the algorithm, but we eventually decided to use the term "machine". This may be a practice that gets adopted more broadly as multiple teams build more structured tasks that are backed by algorithms. Below is how we thought about this decision.
Background
As we build experiences that incorporate augmentation, we are thinking about the terminology to use when referring to suggestions that come from automated systems. If possible, we want to make a smart choice at the outset, to minimize changes and confusion later. For instance, we are looking at sentences in the feature like these:
- "Suggested links are machine-generated, and can be incorrect."
- "Links are recommended by machine, and you will decide whether to add them to the article."
Objectives
We want the terms we use to satisfy these objectives.
- Transparency: users should understand where recommendations come from, and we should be being honest with them.
- Human-in-the-loop: users should understand that their contributions improve recommendations in the future.
- Usability: copy should help users complete the tasks, not confuse or burden them with too much information.
- Consistency: we should use the same copy as much as possible to lower cognitive load.
Terms we considered
Term | Strengths | Weaknesses |
AI |
|
|
Machine |
|
|
Computer |
|
|
Algorithm |
|
|
Automated |
|
|
Methods and findings
- User testing: the Growth team tested "add a link" in English using the terms "artificial intelligence" and "AI". We found that users understood the term well and that English-speaking users understood that they should regard the output of AI with skepticism.
- Experts: we spoke to WMF experts in the fields of artificial intelligence and machine learning. They explained that the link recommendation is not truly "AI", in the way that the term is used in the industry today. They explained that by using that term, we may be over-inflating our work and giving users a false sense of the intelligence of the algorithm. Experts preferred the term "machine", as it would accurately describe the link recommendation algorithm as well as be broad enough to describe almost any other kind of algorithm we might use for structured tasks.
- Multi-lingual community members: we spoke to about seven multilingual colleagues and ambassadors about the terms that would make the most sense in their languages. Not all languages have a short acronym for "AI"; many have long translations. The consensus was that "machine" made good sense in most languages and would be easy to translate.
Result
We are going to use the term "machine" to refer to the link recommendation algorithm, e.g. "Suggested links are machine-generated". See screenshots below to see one of the places where the terminology changed based on this decision.
-
Onboarding screen using the term "AI"
-
Onboarding screen using the term "machine"
Measurement
Hypotheses
The “add a link” workflow structures the process of adding wikilinks to a Wikipedia article, and assists the user with artificial intelligence to point out the clearest opportunities for adding links. Our hypothesis with the “add a link” workflow is that such a structured editing experience will lower the barrier to entry and thereby engage more newcomers, and more kinds of newcomers than an unstructured experience. We further hypothesize that newcomers with the workflow will complete more edits in their first session, and be more likely to return to complete more.
Below are the specific hypotheses we seek to validate. These govern the specifics around which data we'll collect and how we'll analyze it.
- The "add a link" structured task increases our core metrics of activation, retention, and productivity.
- “Add a link” edits are more likely to be successful than unstructured suggested edits, meaning that a user completes the task and saves the edit. They are also more likely to be constructive, meaning that the edit was not reverted, than unstructured suggested edits.
- Users seem to understand this task more than unstructured tasks.
- Users who start with "add a link" will move on to other kinds of tasks, instead of staying siloed (the latter being a primary community concern).
- The perceived quality of the link recommendation algorithm will be high, both from the users who make "add a link" edits and the communities who review those edits.
- Users who get “add a link” and who primarily use/edit wikis on mobile see a larger increase in the effects on retention and productivity relative to desktop users.
Experiment Plan
A randomly selected half of users who get the Growth features will get "add a link" tasks, and the other randomly selected half will get unstructured link tasks. We prefer to give users maximum exposure to these tasks and will therefore not give these users any copyedit tasks by default. In other words, for the purposes of this experiment, we’ll change the default difficulty filters from “links” and “copyedit” to just “links”. For wikis that don’t have unstructured link tasks, all those users get “add a link” and in that case we’ll exclude that wiki from the experiment.
We plan to continue to have a Control group that does not get access to the Growth features, which is a randomly selected 20% of new registrations.
- Group A: users get “add a link” as their only default task type.
- Group B: users get unstructured link task as their only default task type.
- Group C: control (no Growth features)
The experiment will run for a limited time, most likely between four to eight weeks. In practice the experiment will start with our four pilot wikis. After two weeks, we will analyze the leading indicators below to decide whether to extend the experiment to the rest of the Growth wikis.
Experiment Analysis and Findings
After completion of "Add a link" Experiment Analysis, we can conclude that the "add a link" structured task improves outcomes for newcomers over both a control group that did not have access to the Growth features as well as the group that had the unstructured "add links" tasks, particularly when it comes to constructive (non-reverted) edits. The most important points are:
- Newcomers who get the Add a Link structured task are more likely to be activated (i.e. make a constructive first article edit).
- They are also more likely to be retained (i.e. come back and make another constructive article edit on a different day).
- The feature also increases edit volume (i.e. the number of constructive edits made across the first couple weeks), while at the same time improving edit quality (i.e. the likelihood that the newcomer's edits are reverted).
Leading Indicators and Plan of Action
We are at this point fairly certain that Growth features are not detrimental to the wiki communities. That being said, we also want to be careful when experimenting with new features. Therefore, we define a set of leading indicators that we will keep track of during the early stages of the experiment. Each leading indicator comes with a plan of action in case the defined threshold is reached, so that the team knows what to do.
Indicator | Plan of Action |
---|---|
Revert rate | This suggests that the community finds the Add a Link edits to be unconstructive. If the revert rate for Add a Link is significantly higher than that of unstructured link tasks, we will analyze the reverts in order to understand what causes this increase, then adjust the task in order to reduce the likelihood of edits being reverted. |
User rejection rate | This can indicate that we are suggesting a lot of links that are not good matches. If the rejection rate is above 30%, we will QA the link recommendation algorithm and adjust thresholds or make changes to improve the quality of the recommendations. |
Task completion rate | This might indicate that there’s an issue with the editing workflow. If the proportion of users who start the Add a Link task and complete it is lower than 75%, we investigate where in the workflow users leave and deploy design changes to enable them to continue. |
Analysis and Findings
We collected data on usage of Add a Link from deployment on May 27, 2021 until June 14, 2021. This dataset excluded known test accounts, and does not contain data from users who block event logging (e.g. through their ad blocker).
This analysis categorizes users into one of two categories based on when they registered. Those who registered prior to feature deployment on May 27, 2021 are labelled "pre-deployment", and those who registered after deployment are labelled "post-deployment". We do this because users in the "post-deployment" group are randomly assigned (with 50% probability) into either getting Add a Link or the unstructured link task. Users in the "pre-deployment" group have the unstructured link task replaced by Add a Link. By splitting into these two categories, we're able to make meaningful comparisons between Add a Link and the unstructured link task, for example when it comes to revert rate.
Revert rate: We use edit tags to identify edits and reverts, and reverts have to be done within 48 hours of the edit. The latter is in line with common practices for reverts.
User registration | Task type | N edits | N reverts | Revert rate |
---|---|---|---|---|
Post-deployment | Add a Link | 290 | 28 | 9.7% |
Unstructured | 63 | 22 | 34.9% | |
Pre-deployment | Add a Link | 958 | 49 | 5.1% |
For the post-deployment group, a Chi-squared test of proportions finds the difference in revert rate to be statistically significant (). This means that the threshold described in the leading indicator table is not met.
Rejection rate: We define an "edit session" as reaching the edit summary or skip all dialogue, at which point we count the number of links that were accepted, rejected, or skipped. Users can reach this dialogue multiple times, because we think that choosing to go back and review links again is a reasonable choice.
User registration | N accepted | % | N rejected | % | N skipped | % | N total |
---|---|---|---|---|---|---|---|
Post-deployment | 597 | 72.4 | 125 | 15.2 | 103 | 12.5 | 825 |
Pre-deployment | 1,464 | 65.1 | 595 | 26.5 | 189 | 8.4 | 2,248 |
2,061 | 67.1 | 720 | 23.4 | 292 | 9.5 | 3,073 |
The threshold in the leading indicator table was a rejection rate of 30%, and this threshold has not been met.
Over-acceptance rate: This was not part of the original leading indicators, but we ended up checking for it as well in order to understand whether users were clicking "accept" on all the links and saving those edits. We reuse the concept of an "edit session" from the rejection rate analysis, and count the number of users who only have sessions where they accepted all links. In order to understand whether these users make many edits, we measure this for all users as well as for those with five or more edit sessions. In the table below, the "N total" column shows the total number of users with that number of edit sessions, and "N accepted all" the number of users who only have edit sessions where they accepted all suggested links.
User registration | N total | N accepted all | % | |
---|---|---|---|---|
Post-deployment | ≥1 edit | 96 | 31 | 32.3 |
≥5 edits | 6 | 0 | 0.0 | |
Pre-deployment | ≥1 edit | 64 | 10 | 15.6 |
≥5 edits | 19 | 1 | 5.3 |
We find that some users only have sessions where they accepted all links, but these users do not typically continue to make Add a Link edits. Instead, users who make additional edits start rejecting or skipping links as needed.
Task completion rate: We define "starting a task" as having an impression of "machine suggestions mode". In other words, the user is loading the editor with an Add a Link task. "Completing a task" is defined as clicking to save an edit, or confirming that all suggested links were skipped.
User registration | N Started a Task | N Completed 1+ Tasks | % |
---|---|---|---|
Post-deployment | 178 | 96 | 53.9 |
Pre-deployment | 101 | 64 | 63.4 |
279 | 160 | 57.3 |
The threshold defined in the leading indicator table is "lower than 75%", and this threshold has been met. In this case, we're planning to do follow-up analysis to understand more about the tasks, e.g. if they had a low number of suggested links, or if this happens on specific wikis or platforms.
Rejection Reasons
We've analyzed data on why users reject suggested links, focusing on newcomers to help us understand how they learn what constitutes good links in Wikipedia. In this analysis, we used rejections from January and February 2022, and restricted it to actions made within 7 or 28 days since registration. There was no significant difference in patterns between the two, and the data reported here uses the 28 day window. The data was split by wiki, platform (desktop or mobile) and bucketed by the number of Add a Link edits the user had made. We used a logarithmic bucketing scheme with 2 as the base, because that gives us a fair number of buckets early in a user's life while at the same time being easy to understand since the limits double each time.
Platform | Rejection reason | N | % |
---|---|---|---|
Desktop | Almost everyone knows what it is | 2,732 | 53.0% |
Linking to wrong article | 1,377 | 26.7% | |
Other | 688 | 13.0% | |
Text should include more or fewer words | 378 | 7.3% | |
Mobile | Almost everyone knows what it is | 1,835 | 53.3% |
Linking to wrong article | 791 | 23.0% | |
Other | 484 | 14.1% | |
Text should include more or fewer words | 271 | 7.9% | |
Undefined | 62 | 1.8% |
The distribution of these reasons is generally the same across all wikis, platforms, and number of Add a Link edits made. For some combinations of these features, we run into the issue of having few data points available (e.g. because some wikis lean strongly to usage of one platform) and that might result in a somewhat different distribution (e.g. just ones marked "Text should include more or fewer words"). In general, we have a lot of data for users with few edits as that's what most users are.
One thing we do appear to see is that for some wikis the usage of "Other" decreases as the number of Add a Link edits made increases. We interpret this to mean that "Other" might be a catchall/safe category for less experienced users, and that as they become more experienced and confident in labelling a link they'll use a different category.
Engineering
Link recommendation algorithm
See this page for an explanation of the link recommendation algorithm and for statistics around its accuracy. In short, we believe that users will experience an accuracy around 75%, meaning that 75% of the suggestions they get should be added. It is possible to tune this number, but the higher the accuracy is, the fewer candidate link we will be able to recommend. After the feature is deployed, we can look at revert rates to get a sense of how to tune that parameter.
For a detailed understanding of how the algorithm functions and is evaluated, see this research paper.
Link recommendation service backend
To follow along with engineering progress on the backend "add link" service, please see this page on Wikitech.
Articles selection
Articles are selected based on the topic(s) the use chooses. Then articles with a low ratio of links compared to the number of words are selected. A small amount of randomness was added to it so that not every article suggested look the same. The formula is documented on Phabricator.
Deployment
On May 27, 2021, we deployed the first iteration of this task to our four pilot wikis: Arabic, Czech, Vietnamese, and Bengali Wikipedias. It is available to half of new accounts, as described above. All accounts created before the deployment will also have the feature available. After two weeks, we will analyze our leading indicators to determine if any quick changes need to be made. After about four weeks, we will use data and community feedback to determine whether and how to deploy the feature to more wikis.
Since the initial deployment to our pilot wikis, the Growth team has gathered extensive community feedback and data about the usage and value of Add a Link. We then used those learnings to make improvements to the feature so that newcomers would have a better experience and experienced editors would see higher quality edits. We have completed the improvements, and communities are now using iteration 2 of Add a Link.
The deployment to all Wikipedias was completed at the end of 2023, with only few wikis missing: German and English as they require specific community engagement, and very small Wikipedias where there is no critical mass to have enough links.